Hello everyone! We are very excited to introduce CHI NL Meet guest, Prof. Nava Tintarev, where she shares with us her bio and aspirations! 🙂
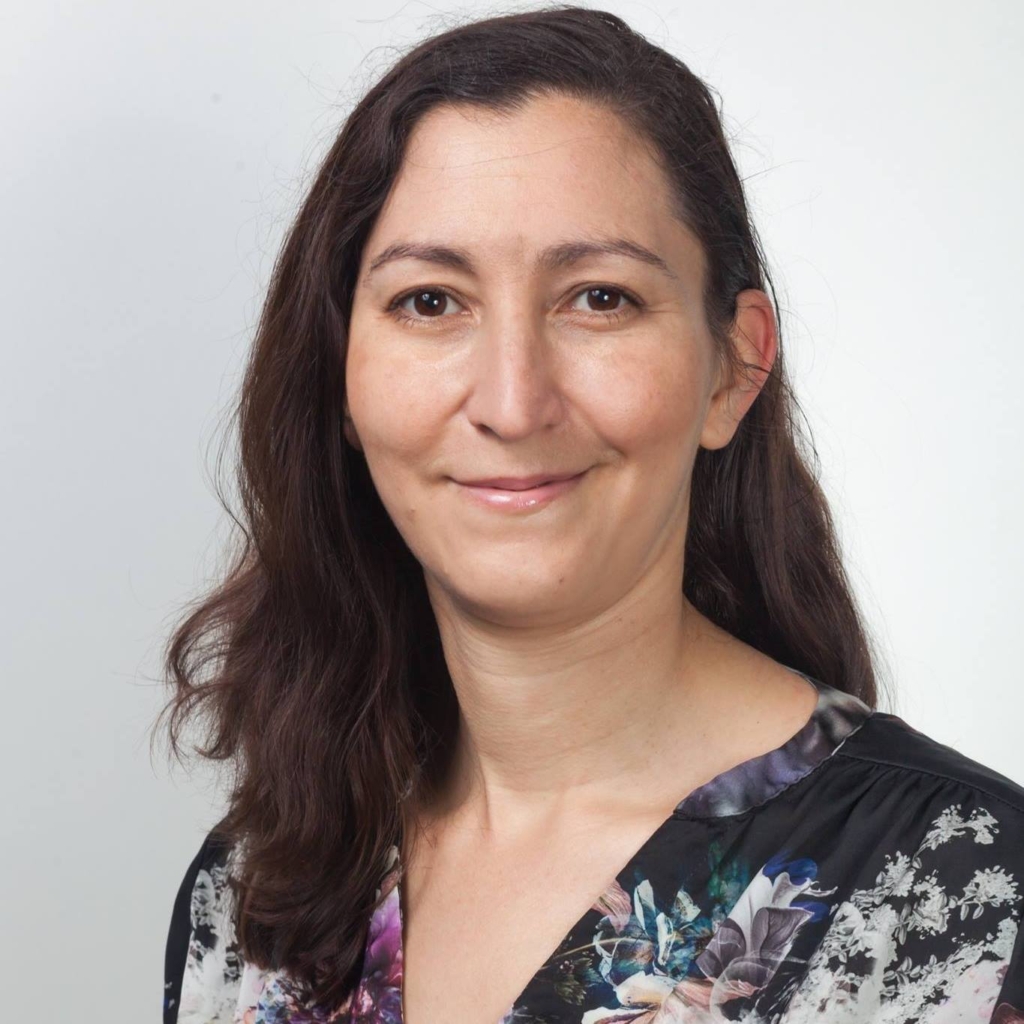
What is your name?
Hi, I’m Professor Nava Tintarev.
What’s your current job / occupation?
I am a Full Professor in Explainable AI at Maastricht University, and a visiting Professor at TU Delft.
And what are you currently working on?
In a way I’m doing what I started doing in my PhD in 2006: developing research and education around human-centered explainable artificial intelligence (XAI). However I seem to be involved more in strategic initiatives now. I am part of the team developing the AI strategy at Maastricht University, including the theme of human-centered AI in the new AI-hub Brightlands. Nationally, I’m a co-Investigator in the national ROBUST program, a ten-year AI research programme focusing on trustworthy AI, where I am the chair of the social sciences and humanities committee. By mixing talent working on algorithmic advances with talent working on societal embedding and uptake of technology, we aim to improve our algorithm development process and increase our potential for impact and achieving the sustainable development goals.
However, what I love the most is designing innovative user studies and experiment methodologies, so I am still heavily involved in writing many papers on explainable search and recommendations. 🙂
What is your proudest achievement so far? Can be anything, professional or otherwise.
I am the most proud of the impact I’ve had on the students I’ve taught and supervised. Developing and growing the next generation of talent is a privilege, and this kind of impact can leave a mark on both research and practice, long after I and the papers that I wrote become obsolete. I am also proud that I became a full professor before turning 40, I was not expecting that!
Which person, paper, or concept has had the biggest influence on your work?
Almost everyone I’ve worked with has added a dimension to how I look at problems, questioned one of my underlying assumptions, or given me a new tool for my toolbox. I really think the most important thing is to keep learning and keep questioning assumptions. Early on, my two PhD advisors shaped the way I approach problems: Ehud Reiter taught me the value of always trying systems out with people, and Judith Masthoff taught me how to focus my thinking and to translate it into a precise experimental design. In Aberdeen I learned a lot about Natural Language Generation and adaptive systems, and my research visit to UCSB (California, USA) with the Four Eyes lab as a postdoc deepened my interest in interactive systems and explanations.
What breakthroughs or developments do you expect in your profession in the next 10 years?
I already see an increased focus on responsible and human-centered AI, and I think this need is genuine and pressing. On the more positive side, I think it’s likely that different forms of augmented or hybrid intelligence will become the norm, e.g., in complex or uncertain decision-making scenarios. Certainly the recent developments in games such as Go, large language models, and computational creativity point in this direction. As part of that I expect that we’ll become better at measuring and evaluating how effective these “collaborations” are over longer durations.
What have you recently read, watched, or listened to that you would like to recommend to others?
Since I became a full Prof and became responsible for guiding more people, I started reading more leadership books. I really like Amy C. Edmonson’s “The Fearless Organization”. Main takeaway: More productive teams also register more mistakes. Not because they are more error-prone, but because people raise issues when or before they occur. The old style of managing does not work in the context of the creative economy which is fast-changing, and the value of a diverse team only shines through when people feel safe and free to speak up. Recently, I’m finding the Women at Work from Harvard Business Review indispensable. It has top notch advice for leaders regardless of gender, but of course some issues that are more typical for women, on average.
What or who got you interested in the area of Human-Computer Interaction / User Experience, and why?
Soon after starting my bachelor’s in computer science, I had the idea that I needed to understand human psychology and cognition in order to design good software. Studying psychology as part of my undergraduate electives, and then pursuing a Master’s degree which was effectively human-computer interaction (well, automatically personalizing text to motivate students) just helped cement that.
Finally, a chance for self-promotion: what should we read, watch, or listen to so we learn more about your work?
There’s the papers that have the largest number of citations, but I’d like to showcase some of our more recent work and give exposure to the upcoming generation of scientists. With Federico Cau and others we have been looking at how AI/user confidence interact with over- and under-reliance on explanations. We looked at this for difference styles of logical reasoning. So, the underlying deep neural network is the same, but the explanation “models” are different. Among other findings, inductive style explanations (using examples of similar instances) led to over-reliance on the AI advice in three domains – it was the most persuasive explanation style, even when the AI was incorrect. These are the upcoming papers:
- Cau, F. M., Hauptmann, H., Spano, L. D., & Tintarev, N. (2023, to appear). Supporting High-Uncertainty Decisions through AI and Logic-Style Explanations. IUI.
- Cau, F. M., Hauptmann, H., Spano, L. D., & Tintarev, N. (2023, to appear) Effects of AI and Logic-Style Explanations on Users’ Decisions under Different Levels of Uncertainty. ACM Transactions on Interactive Intelligent Systems (TiiS). DOI: https://dl.acm.org/doi/10.1145/3588320
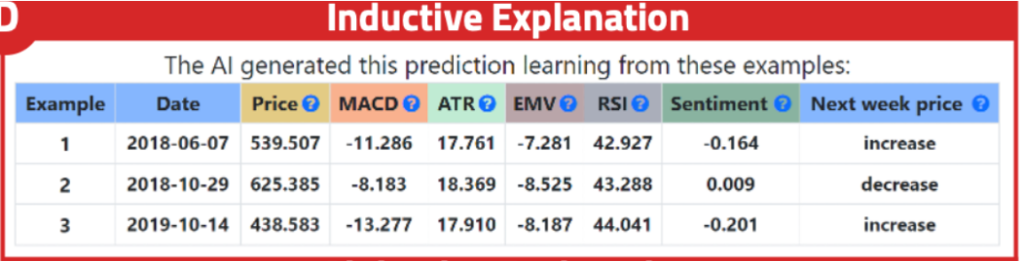
With Tim Draws, Alisa Rieger, and others, we have been looking at developing the groundwork to mitigate confirmation bias when people are searching about disputed topics. We have been looking at, among others, how to explain the stance of search results, and how the predictive model and explanation model interact, as well as which search results people actually interact with. Here are some good starting points:
- Draws, T., Ramamurthy, K. N., Soares, I. B., Dhurandhar, A., Padhi, I., Timmermans, B., & Tintarev, N. (2023, to appear). Explainable Cross-Topic Stance Detection for Search Results. CHIIR.
- Rieger, Alisa, et al. “This Item Might Reinforce Your Opinion: Obfuscation and Labeling of Search Results to Mitigate Confirmation Bias.” Proceedings of the 32nd ACM Conference on Hypertext and Social Media. 2021.
- Draws, Tim, et al. “This Is Not What We Ordered: Exploring Why Biased Search Result Rankings Affect User Attitudes on Debated Topics.” Proceedings of the 44th International ACM SIGIR Conference on Research and Development in Information Retrieval. 2021.
CHI NL Meet is a regular feature on the CHI NL blog, where board members and blog editors Lisa and Abdo invite a CHI NL member to introduce themselves to the wider SIGCHI community and world 🌍.
✨ Get updates about HCI activities in the Netherlands ✨
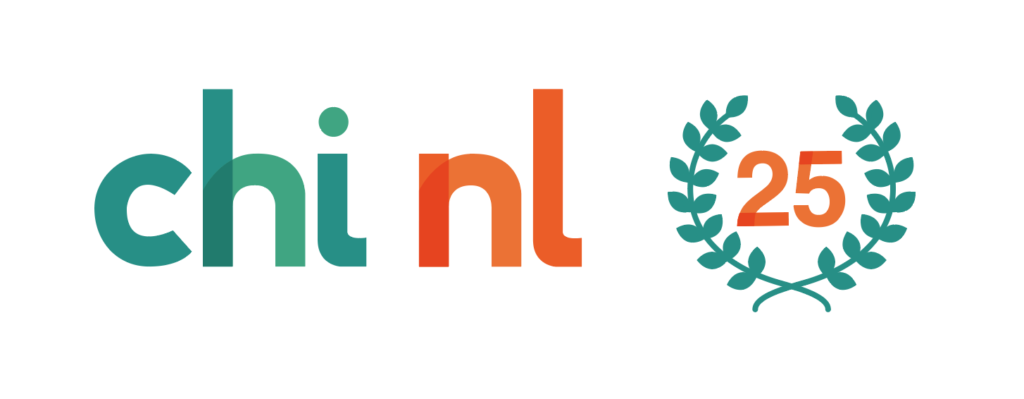
CHI Nederland (CHI NL) is celebrating its 25th year anniversary this year, and we have much in store to acknowledge this occasion. Stay tuned!